What do the next 20 years hold for artificial intelligence?
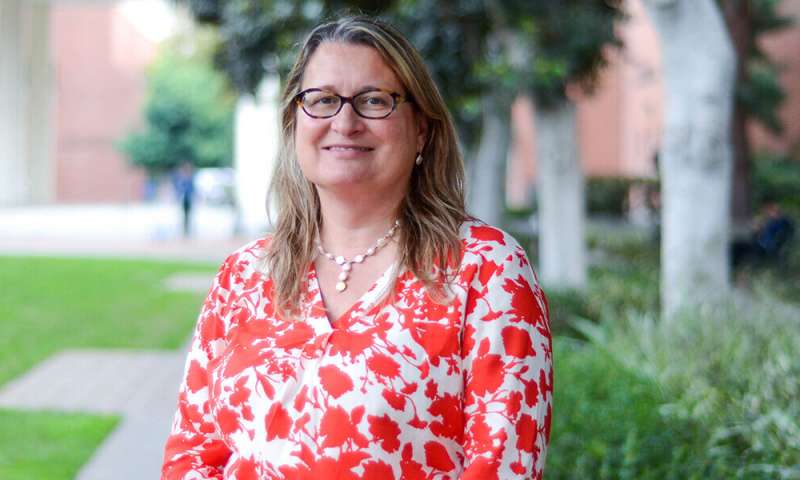
Yolanda Gil, president of the Association for the Advancement of Artificial Intelligence (AAAI), discusses what it will take to move AI forward without moving safety backward.
The year is 2031. An outbreak of a highly contagious mosquito-borne virus in the U.S. has spread quickly to major cities around the world. It’s all hands on deck to stop the disease from spreading–and that includes the deployment of artificial intelligence (AI) systems, which scour online news and social media for relevant data and patterns.
Working with these results, and data gathered from numerous hospitals around the world, scientists discover an interesting link to a rare neurological condition and a treatment is developed. Within days, the disease is under control. It’s not hard to imagine this scenario—but whether future AI systems will be competent enough to do the job depends in large part on how we tackle AI development today.
That’s according to a new 20-year Artificial Intelligence Roadmap co-authored by Yolanda Gil, a USC computer science research professor and research director at the USC Viterbi Information Sciences Institute (ISI), with computer science experts from universities across the U.S.
Recently published by the Computing Community Consortium, funded by the National Science Foundation, the roadmap aims to identify challenges and opportunities in the AI landscape, and to inform future decisions, policies and investments in this area.
As president of the Association for the Advancement of Artificial Intelligence (AAAI), Gil co-chaired the roadmap with Bart Selman, a computer science professor at Cornell University.
We spoke with Gil about what AI means today, what it will take to build more intelligent and competent AI in the future, and how to ensure AI operates safely as it approaches humans in its intelligence.
The interview has been condensed and edited for clarity.
Why did you undertake the AI Roadmap effort with the Computing Community Consortium?
We really wanted to highlight what it will take for AI systems to become more intelligent in the long-term. So, you think of conversational interfaces like Siri and Alexa—even today, they still have a lot of limitations. What would it take to make them AI systems more aware of our world? For instance, for them to understand “What is a mother?” and “Why it is important to remind me about my mother’s birthday?” Those are the kinds of questions we are asking in the report.
We wanted to understand what research is needed for our AI systems—the conversational interfaces, the self-driving cars, the robots—to have additional capabilities. If we don’t invest in longer-term areas of research, there may not be a next generation of systems that will understand what our world is about, that will be better at learning about their tasks, and that will be more competent.
What does the phrase artificial intelligence actually mean to you in 2019?
AI is really about studying and creating capabilities that we typically associate with intelligent behaviors. These tend to be related to the mind, intelligence and thought, as opposed to more small-scale reactive behaviors.
We usually think about intelligence in terms of capabilities that involve thinking, reasoning, learning; in terms of managing information and complex tasks that affect the world around us. Things like, can you learn when you have gone through a lot of experiences? Or examples? Can you learn from observing somebody doing a task? Can you learn from your own mistakes? Can you learn from being explained how something works?
Learning is just one aspect of AI. There are also other aspects that have to do with reasoning, planning and organizing. And then other parts of AI related to natural language and communicating, and others related to robotics.
So, there are a lot of different intelligent behaviors that we include under the umbrella of AI. Given that we have lots of AI systems around us, a key question is: How do we elevate them to have the next generation of capabilities?
Are AI researchers really trying to emulate human thought? Or is machine intelligence something completely different?
Well, a lot of research is looking at human behavior as an inspiration for AI, or as a target, by trying to model human intelligence and human behavior. But that’s just one sector of the community.
There are other researchers, like me, that look at human behavior and use it as a motivation for creating or engineering machines that “think,” without regards to how human memory works, or what cognitive experiments tell us about human thought, or human biology or the brain. So, we take more of an engineering approach.
And sometimes you see AI that touches on both—so you will have truly human-inspired cognitive systems that approach intelligent tasks the way humans would do them. For example, some robots are trying to appear human, but a lot of other robots will just do what task and you know, it doesn’t matter what they look like. Research is progressing across both areas.
What do you find particularly impressive in current AI research?
Seeing the success that these systems are having in important applications like medicine and other areas of science—that’s very exciting to me. AI systems have been used in medicine for decades now, but they were very complex and time-consuming to build, and they would only have acceptable performance in certain areas. I think now we’re seeing AI systems penetrating new areas of medicine. For example, AI systems are very good at identifying tumors or certain types of cells based on pathology images.
What big challenges do you think need to be overcome to move the needle in AI?
The report highlights many challenges organized into three major research areas. One big challenge is integrating intelligence capabilities. Right now, for instance, you have robots that vacuum, you have AI systems that talk, but it’s very hard to integrate those separate capabilities to work together.
The second is communication: how does AI connect with humans and convey information. Today, we converse with AI systems, but there are no stakes in the conversation, so misunderstandings are accepted, and a productive result is desirable, but not crucial. But what if those things really mattered?
The third is self-aware learning, so for example, what would it take for an AI to think: “I shouldn’t use what I’ve learned because I haven’t seen enough examples of it yet” or “given the few examples I have seen, I should analyze them in new ways to get more information out of them.” We don’t have systems that can do that yet.
These questions present a very ambitious and exciting research agenda for AI in the next 20 years.
What has to change for AI research to make greater strides?
The findings from the report indicate that, to pursue this research agenda, we need to expand a lot of the current university infrastructure for AI. We need to move into an era where there are more substantial academic collaborations on AI problems, and more substantial resources such as hardware, data resources and open software toolkits.
As inspiration, we’re pointing to billion-dollar efforts that made a significant difference in the world: The Human Genome Project, which really propelled the field of genomics; or the LIGO project, which led to the experimental observation of gravitational waves. What we’re saying is unless we are at that level of investment, it will take a very long time to get to the next level of AI capabilities.
In the United States, we have many fantastic researchers and the best universities. I think that we need to continue to support individual research projects as we have in the past, but we need to add a significant new layer of much larger efforts. That is why the report recommends the creation of multi-decadal, multi-university research centers that will take on big questions and large organizations devoted to specific problems.
What are you most excited about over the next 20 years in AI?
I think the application of AI for scientific research and discovery has the potential to really change the world, and this is the focus of my research. There are many challenges in terms of representing scientific knowledge in a machine-readable way, to integrate AI systems as part of the research process. So, empowering scientists with better tools is a really exciting area for me. My dream is that in 20 years, a scientist will come into the office in the morning and their AI system will tell them about interesting results it worked on overnight. We will be able to make discoveries at a faster rate, from finding cures for diseases to better managing natural resources, such as water.
The road ahead looks exciting, to say the least, but how do we ensure people are not left out as AI moves forward?
We need to ensure that there are fair opportunities for everyone to access this technology. We have to push AI to the kindergarten level, giving kids an opportunity to understand how this technology can impact their lives, all the way through to college.
In the report, we recommend careers for AI engineers not only at doctoral level, but at all levels, including bachelor’s degrees and even high school diplomas in AI. We need technicians to repair robots, to prepare data for AI systems, and to use AI tools in new application areas.
Are people justified in their concerns about AI?
I think we have to be cognizant that when AI is deployed in real life in particular sectors, it creates new challenges for security, trust, and ethics. My first concern is the humans who deploy and operate AI systems, rather than the AI system itself —that’s why I would like to see more engagement in policy and ethical uses of AI.
Today, many AI deployments are not going through a stage of safety engineering and ethical thinking about that particular use of the technology. So, I think we should put a lot more investment in that. In the report, we recommend the creation of new degrees and career paths explicitly on AI ethics and safety in AI engineering.
It’s also important to note that these issues are not just the AI researchers’ problem. AI research has so many ramifications and so many connections to every discipline. AI researchers are genuinely excited to engage with other communities. We hope the report will help to foster this dialogue across disciplines and communities, at USC and beyond.
What do you think is the greatest myth about AI?
I think humans ascribe intelligence to AI very generously. We interact with an AI system, and we start to imagine that it is really understanding us, just because it said “hello.” But in reality, it didn’t really understand anything. AI systems are often perceived as being more capable than they actually are. So, when you use or interact with an AI system, use some critical thinking about what it is really capable of at this point in time.