Machine learning predicts behavior of biological circuits
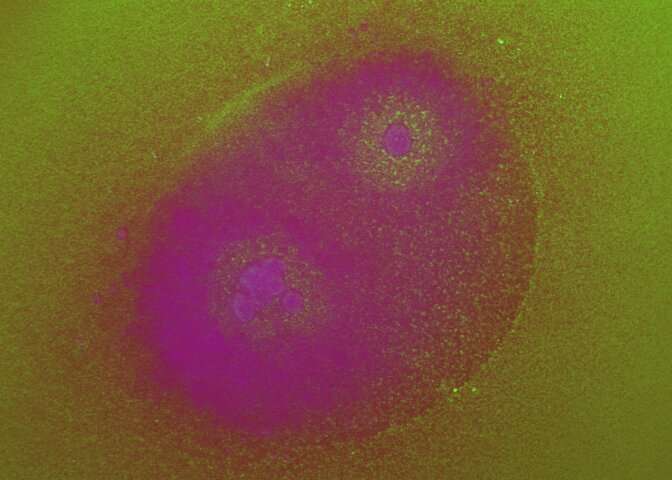
Biomedical engineers at Duke University have devised a machine learning approach to modeling the interactions between complex variables in engineered bacteria that would otherwise be too cumbersome to predict. Their algorithms are generalizable to many kinds of biological systems.
In the new study, the researchers trained a neural network to predict the circular patterns that would be created by a biological circuit embedded into a bacterial culture. The system worked 30,000 times faster than the existing computational model.
To further improve accuracy, the team devised a method for retraining the machine learning model multiple times to compare their answers. Then they used it to solve a second biological system that is computationally demanding in a different way, showing the algorithm can work for disparate challenges.
The results appear online on September 25 in the journal Nature Communications.
“This work was inspired by Google showing that neural networks could learn to beat a human in the board game Go,” said Lingchong You, professor of biomedical engineering at Duke.
“Even though the game has simple rules, there are far too many possibilities for a computer to calculate the best next option deterministically,” You said. “I wondered if such an approach could be useful in coping with certain aspects of biological complexity confronting us.”
The challenge facing You and his postdoctoral associate Shangying Wang was determining what set of parameters could produce a specific pattern in a bacteria culture following an engineered gene circuit.
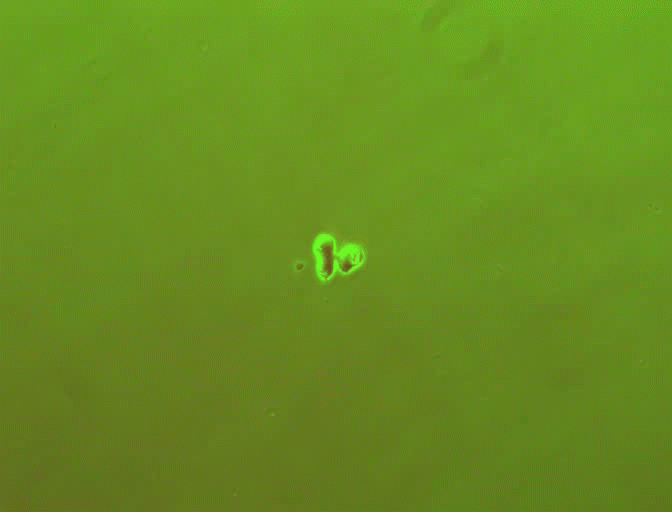
In previous work, You’s laboratory programmed bacteria to produce proteins that, depending on the specifics of the culture’s growth, interact with one another to form rings. By controlling variables such as the size of the growth environment and the amount of nutrients provided, the researchers found they could control the ring’s thickness, how long it took to appear and other characteristics.
By changing any number of dozens of potential variables, the researchers discovered they could do more, such as causing the formation of two or even three rings. But because a single computer simulation took five minutes, it became impractical to search any large design space for a specific result.
For their study, the system consisted of 13 bacterial variables such as the rates of growth, diffusion, protein degradation and cellular movement. Just to calculate six values per parameter would take a single computer more than 600 years. Running it on a parallel computer cluster with hundreds of nodes might cut that run-time down to several months, but machine learning can cut it down to hours.
“The model we use is slow because it has to take into account intermediate steps in time at a small enough rate to be accurate,” said You. “But we don’t always care about the intermediate steps. We just want the end results for certain applications. And we can (go back to) figure out the intermediate steps if we find the end results interesting.”
To skip to the end results, Wang turned to a machine learning model called a deep neural network that can effectively make predictions orders of magnitude faster than the original model. The network takes model variables as its input, initially assigns random weights and biases, and spits out a prediction of what pattern the bacterial colony will form, completely skipping the intermediate steps leading to the final pattern.
While the initial result isn’t anywhere close to the correct answer, the weights and biases can be tweaked each time as new training data are fed into the network. Given a large enough “training” set, the neural network will eventually learn to make accurate predictions almost every time.
To handle the few instances where the machine learning gets it wrong, You and Wang came up with a way to quickly check their work. For each neural network, the learning process has an element of randomness. In other words, it will never learn the same way twice, even if it’s trained on the same set of answers.
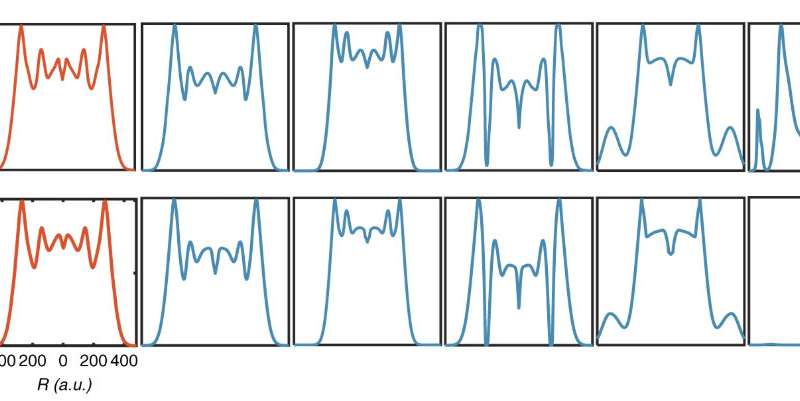
The researchers trained four separate neural networks and compared their answers for each instance. They found that when the trained neural networks make similar predictions, these predictions were close to the right answer.
“We discovered we didn’t have to validate each answer with the slower standard computational model,” said You. “We essentially used the ‘wisdom of the crowd’ instead.”
With the machine learning model trained and corroborated, the researchers set out to use it to make new discoveries about their biological circuit. In the initial 100,000 data simulations used to train the neural network, only one produced a bacterial colony with three rings. But with the speed of the neural network, You and Wang were not only able to find many more triplets, but determine which variables were crucial in producing them.
“The neural net was able to find patterns and interactions between the variables that would have been otherwise impossible to uncover,” said Wang.
As a finale to their study, You and Wang tried their approach on a biologic system that operates randomly. Solving such systems requires a computer model to repeat the same parameters many times to find the most probable outcome. While this is a completely different reason for long computational run times than their initial model, the researchers found their approach still worked, showing it is generalizable to many different complex biological systems.
The researchers are now trying to use their new approach on more complex biological systems. Besides running it on computers with faster GPUs, they’re trying to program the algorithm to be as efficient as possible.
“We trained the neural network with 100,000 data sets, but that might have been overkill,” said Wang. “We’re developing an algorithm where the neural network can interact with simulations in real-time to help speed things up.”
“Our first goal was a relatively simple system,” said You. “Now we want to improve these neural network systems to provide a window into the underlying dynamics of more complex biological circuits.”